*免责声明:
1\此方法仅提供参考
2\搬了其他博主的操作方法,以贴上路径.
3*
场景一: 使用conda pack进行打包个人项目
场景二:
…
场景一: 使用conda pack进行打包个人项目
1.1 导出包列表
activate jiance
pip list --format=freeze >requirements.txt
1.2 打包 yolo-smoke项目,支持pip install 的安装形式
- 在 requirements.txt 的同级目录下创建 setup.py , 根据需求修改对应的值,内容大致如下:
#!/usr/bin/env python
# Copyright (c) OpenMMLab. All rights reserved.
import os
import os.path as osp
import platform
import shutil
import sys
import warnings
from setuptools import find_packages, setup
import torch
from torch.utils.cpp_extension import (BuildExtension, CppExtension,
CUDAExtension)
def readme():
with open('README.md', encoding='utf-8') as f:
content = f.read()
return content
version_file = 'yolo-smoke/version.py' # 可以参考 mmdetetction工程的下的version文件
# 获取代码的版本号
def get_version():
with open(version_file, 'r') as f:
exec(compile(f.read(), version_file, 'exec'))
return locals()['__version__']
def make_cuda_ext(name, module, sources, sources_cuda=[]):
define_macros = []
extra_compile_args = {'cxx': []}
if torch.cuda.is_available() or os.getenv('FORCE_CUDA', '0') == '1':
define_macros += [('WITH_CUDA', None)]
extension = CUDAExtension
extra_compile_args['nvcc'] = [
'-D__CUDA_NO_HALF_OPERATORS__',
'-D__CUDA_NO_HALF_CONVERSIONS__',
'-D__CUDA_NO_HALF2_OPERATORS__',
]
sources += sources_cuda
else:
print(f'Compiling {name} without CUDA')
extension = CppExtension
return extension(
name=f'{module}.{name}',
sources=[os.path.join(*module.split('.'), p) for p in sources],
define_macros=define_macros,
extra_compile_args=extra_compile_args)
def parse_requirements(fname='requirements.txt', with_version=True):
"""Parse the package dependencies listed in a requirements file but strips
specific versioning information.
Args:
fname (str): path to requirements file
with_version (bool, default=False): if True include version specs
Returns:
List[str]: list of requirements items
CommandLine:
python -c "import setup; print(setup.parse_requirements())"
"""
import re
import sys
from os.path import exists
require_fpath = fname
def parse_line(line):
"""Parse information from a line in a requirements text file."""
if line.startswith('-r '):
# Allow specifying requirements in other files
target = line.split(' ')[1]
for info in parse_require_file(target):
yield info
else:
info = {'line': line}
if line.startswith('-e '):
info['package'] = line.split('#egg=')[1]
elif '@git+' in line:
info['package'] = line
else:
# Remove versioning from the package
pat = '(' + '|'.join(['>=', '==', '>']) + ')'
parts = re.split(pat, line, maxsplit=1)
parts = [p.strip() for p in parts]
info['package'] = parts[0]
if len(parts) > 1:
op, rest = parts[1:]
if ';' in rest:
# Handle platform specific dependencies
# http://setuptools.readthedocs.io/en/latest/setuptools.html#declaring-platform-specific-dependencies
version, platform_deps = map(str.strip,
rest.split(';'))
info['platform_deps'] = platform_deps
else:
version = rest # NOQA
info['version'] = (op, version)
yield info
def parse_require_file(fpath):
with open(fpath, 'r') as f:
for line in f.readlines():
line = line.strip()
if line and not line.startswith('#'):
for info in parse_line(line):
yield info
def gen_packages_items():
if exists(require_fpath):
for info in parse_require_file(require_fpath):
parts = [info['package']]
if with_version and 'version' in info:
parts.extend(info['version'])
if not sys.version.startswith('3.4'):
# apparently package_deps are broken in 3.4
platform_deps = info.get('platform_deps')
if platform_deps is not None:
parts.append(';' + platform_deps)
item = ''.join(parts)
yield item
packages = list(gen_packages_items())
return packages
def add_mim_extension():
"""Add extra files that are required to support MIM into the package.
These files will be added by creating a symlink to the originals if the
package is installed in `editable` mode (e.g. pip install -e .), or by
copying from the originals otherwise.
"""
# parse installment mode
if 'develop' in sys.argv:
# installed by `pip install -e .`
if platform.system() == 'Windows':
# set `copy` mode here since symlink fails on Windows.
mode = 'copy'
else:
mode = 'symlink'
elif 'sdist' in sys.argv or 'bdist_wheel' in sys.argv:
# installed by `pip install .`
# or create source distribution by `python setup.py sdist`
mode = 'copy'
else:
return
# filenames = ['tools', 'configs', 'demo', 'model-index.yml']
filenames = ['tests', 'model-index.yml']
repo_path = osp.dirname(__file__)
mim_path = osp.join(repo_path, 'mmdet', '.mim')
os.makedirs(mim_path, exist_ok=True)
for filename in filenames:
if osp.exists(filename):
src_path = osp.join(repo_path, filename)
tar_path = osp.join(mim_path, filename)
if osp.isfile(tar_path) or osp.islink(tar_path):
os.remove(tar_path)
elif osp.isdir(tar_path):
shutil.rmtree(tar_path)
if mode == 'symlink':
src_relpath = osp.relpath(src_path, osp.dirname(tar_path))
os.symlink(src_relpath, tar_path)
elif mode == 'copy':
if osp.isfile(src_path):
shutil.copyfile(src_path, tar_path)
elif osp.isdir(src_path):
shutil.copytree(src_path, tar_path)
else:
warnings.warn(f'Cannot copy file {src_path}.')
else:
raise ValueError(f'Invalid mode {mode}')
if __name__ == '__main__':
add_mim_extension()
setup(
name='yolo-smoke', # 算法打包的名称
version=get_version(), # 获取版本号
description='OpenMMLab Detection Toolbox and Benchmark', # 算法的描述
long_description=None, # long_description=readme(),
long_description_content_type='text/markdown',
author='yourname',
author_email='yourname@gmail.com',
keywords='computer vision, object detection, smoke , yolo',
url='none' , # 项目地址 'https://github.com/open-mmlab/mmdetection',
packages=find_packages(exclude=('docs', 'tests', 'demo')), # 打包需要略过的文件夹名称
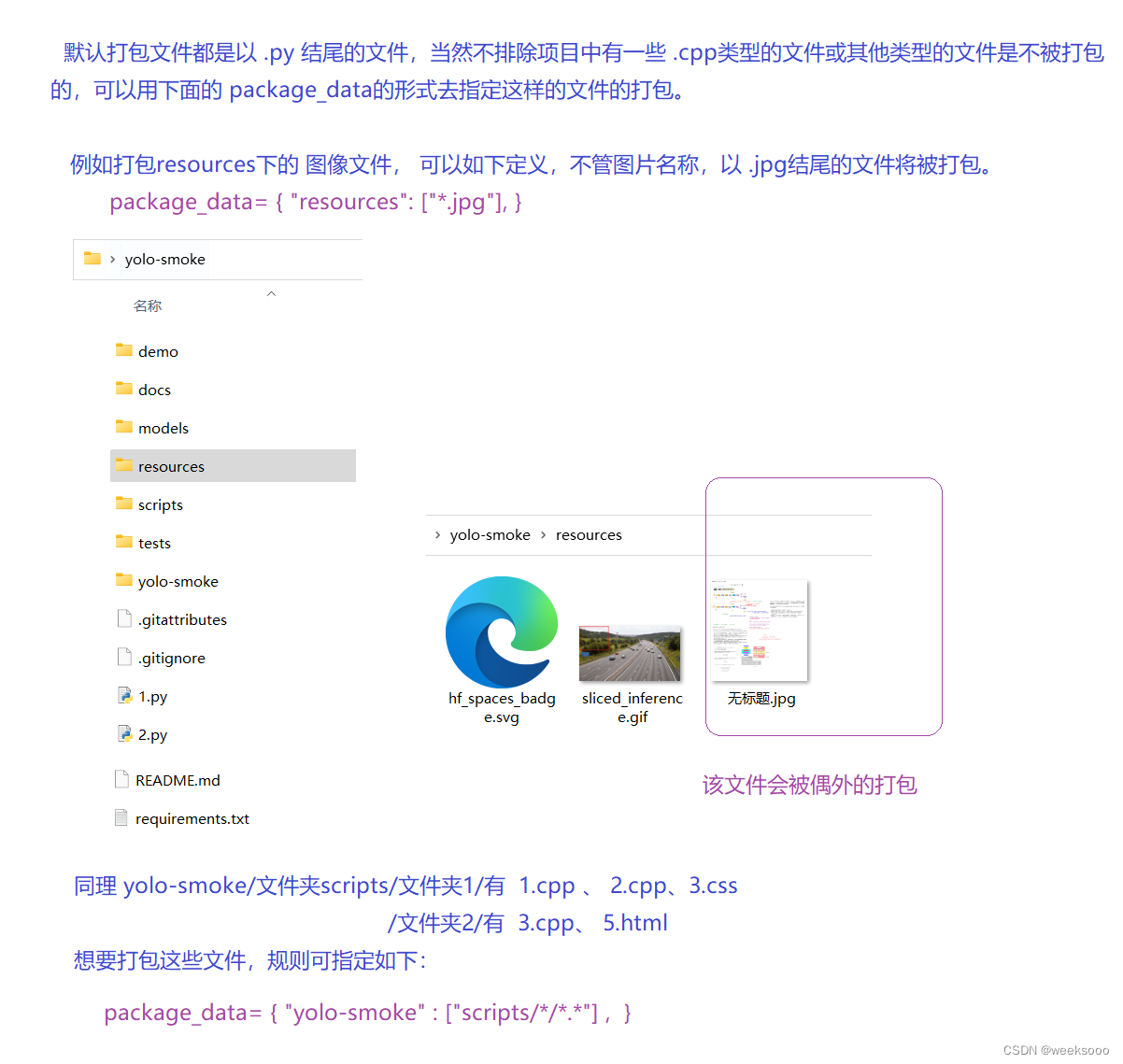
include_package_data=True,
classifiers=[
'Development Status :: 5 - Production/Stable',
'License :: OSI Approved :: Apache Software License',
'Operating System :: OS Independent',
'Programming Language :: Python :: 3',
'Programming Language :: Python :: 3.7',
'Programming Language :: Python :: 3.8',
'Programming Language :: Python :: 3.9',
],
license='Apache License 2.0',
install_requires=parse_requirements('requirements/runtime.txt'),
# extras_require={
# 'all': parse_requirements('requirements.txt'),
# 'tests': parse_requirements('requirements/tests.txt'),
# 'build': parse_requirements('requirements/build.txt'),
# 'optional': parse_requirements('requirements/optional.txt'),
# 'mim': parse_requirements('requirements/mminstall.txt'),
# },
ext_modules=[],
cmdclass={'build_ext': BuildExtension},
zip_safe=False)
-
如果有的文件夹想要参与打包却没有 init.py, 手动的创建该文件。
-
运行 setup.py 文件
python setup.py bdist_wheel
- yolo-smoke 打包测试,在 jiance 的环境下执行以下语句
python
import yolo-smoke
1.3 使用conda pack 打包整个 jiance 环境
- 打开jiance环境的物理位置,如 D:anaconda/envs/ jiance, 切换到上级目录,也就是D:anaconda/envs下 cmd 打开,安装 conda-pack包
D:\Anaconda\envs> pip install conda-pack
- 执行打包语句:
conda pack -n jiance -o yolo_smoke_algorithm.tar.gz --ignore-editable-packages
- 最中会在 anaconda/envs 下生成 yolo_smoke_algorithm.tar.gz 的压缩包。
1.4 项目移植到其他机器上(不想要安装conda/python环境)
- 用 cmd 切换到d盘,创建 yolo_smoke_algorithm 文件夹,将 yolo_smoke_algorithm.tar.gz 复制到 yolo_smoke_algorithm 文件夹下 ,使用命令行的方式解压。
tar -zxvf yolo_smoke_algorithm.tar.gz
方式一:pycharm中使用
- 在pycharm的 File/Settings/project:xxx/Python Interpreter 选择 Add Interpreter,添加到 System Interpreter里面 选择 D:\yolo-smoke_algorithm\python.exe
方式二:anaconda管理
- 命令行的方式解压 yolo_smoke_algorithm.tar.gz 包到 yolo_smoke_algorithm 文件夹下,将该 yolo_smoke_algorithm 文件夹拷贝到 anaconda/envs 下,作为anaconda的一个环境正常的
activate yolo_smoke_algorithm
激活环境。
方式三:命令行指定解释器的方式
D:\yolo_smoke_algorithm\python.exe py文件路径
D:\yolo_smoke_algorithm\python.exe D:\aa\1.py
方式四:
- 在 yolo_smoke_algorithm 文件夹下打开cmd窗口,激活环境
.\Scripts\activate.bat
# 激活环境
D:\yolo_smoke_algorithm> .\Scripts\activate.bat
# 退出环境
(yolo_smoke_algorith)D:\yolo_smoke_algorithm> .\Scripts\deactivate.bat