构建卷积网络
#include<torch/torch.h>
#include<torch/script.h>
#include<iostream>
using std::cout; using std::endl;
class LinearBnReluImpl : public torch::nn::Module
{
private:
torch::nn::Linear ln{ nullptr };
torch::nn::BatchNorm1d bn{ nullptr };
public:
LinearBnReluImpl(int input_features, int out_features);
torch::Tensor forward(torch::Tensor x);
};
TORCH_MODULE(LinearBnRelu);
inline torch::nn::Conv2dOptions conv_options(
int64_t in_planes, int64_t out_planes, int64_t kernel_size,
int64_t stride = 1, int64_t padding = 0, bool with_bias = false
)
{
torch::nn::Conv2dOptions conv_options = torch::nn::Conv2dOptions(in_planes, out_planes, kernel_size);
conv_options.stride(stride);
conv_options.padding(padding);
conv_options.bias(with_bias);
return conv_options;
}
class ConvReluBnImpl : public torch::nn::Module
{
private:
torch::nn::Conv2d conv{ nullptr };
torch::nn::BatchNorm2d bn{ nullptr };
public:
ConvReluBnImpl(int input_channel, int output_channel, int kernel_size, int stride, int padding=1);
torch::Tensor forward(torch::Tensor x);
};
TORCH_MODULE(ConvReluBn);
class MLP : public torch::nn::Module
{
private:
int mid_features[3] = { 32, 64, 128 };
LinearBnRelu ln1{ nullptr };
LinearBnRelu ln2{ nullptr };
LinearBnRelu ln3{ nullptr };
torch::nn::Linear out_ln{ nullptr };
public:
MLP(int in_features, int out_features);
torch::Tensor forward(torch::Tensor x);
};
class plainCNN : public torch::nn::Module
{
private:
int mid_channels[3]{ 32,64,128 };
ConvReluBn conv1{ nullptr };
ConvReluBn down1{ nullptr };
ConvReluBn conv2{ nullptr };
ConvReluBn down2{ nullptr };
ConvReluBn conv3{ nullptr };
ConvReluBn down3{ nullptr };
torch::nn::Conv2d out_conv{ nullptr };
public:
plainCNN(int in_channels, int out_channels);
torch::Tensor forward(torch::Tensor x);
};
int main()
{
plainCNN c(3, 2);
auto x = torch::rand({ 1,3,224,224 }, torch::kFloat);
auto a = c.forward(x);
cout <<"[in Main]: "<< a.sizes() << endl;
return 0;
}
LinearBnReluImpl::LinearBnReluImpl(int input_features, int out_features)
{
ln = register_module("ln", torch::nn::Linear(torch::nn::LinearOptions(input_features, out_features)));
bn = register_module("bn", torch::nn::BatchNorm1d(out_features));
}
torch::Tensor LinearBnReluImpl::forward(torch::Tensor x)
{
x = torch::relu(ln->forward(x));
x = bn(x);
return x;
}
ConvReluBnImpl::ConvReluBnImpl(int input_channel, int output_channel, int kernel_size, int stride, int padding)
{
conv = register_module("conv", torch::nn::Conv2d(conv_options(input_channel, output_channel, kernel_size, stride, padding)));
bn = register_module("bn", torch::nn::BatchNorm2d(output_channel));
}
torch::Tensor ConvReluBnImpl::forward(torch::Tensor x)
{
x = torch::relu(conv->forward(x));
x = bn(x);
return x;
}
MLP::MLP(int in_features, int out_features)
{
ln1 = LinearBnRelu(in_features, mid_features[0]);
ln2 = LinearBnRelu(mid_features[0], mid_features[1]);
ln3 = LinearBnRelu(mid_features[1], mid_features[2]);
out_ln = torch::nn::Linear(mid_features[2], out_features);
ln1 = register_module("ln1", ln1);
ln2 = register_module("ln2", ln2);
ln3 = register_module("ln3", ln3);
out_ln = register_module("out_ln", out_ln);
}
torch::Tensor MLP::forward(torch::Tensor x)
{
x = ln1->forward(x);
x = ln2->forward(x);
x = ln3->forward(x);
x = out_ln->forward(x);
return x;
}
plainCNN::plainCNN(int in_channels, int out_channels)
{
conv1 = ConvReluBn(in_channels, mid_channels[0], 3, 1);
down1 = ConvReluBn(mid_channels[0], mid_channels[0], 3, 2);
conv2 = ConvReluBn(mid_channels[0], mid_channels[1], 3,1);
down2 = ConvReluBn(mid_channels[1], mid_channels[1], 3, 2);
conv3 = ConvReluBn(mid_channels[1], mid_channels[2], 3,1);
down3 = ConvReluBn(mid_channels[2], mid_channels[2], 3, 2);
out_conv = torch::nn::Conv2d(conv_options(mid_channels[2], out_channels, 3));
conv1 = register_module("conv1", conv1);
down1 = register_module("down1", down1);
conv2 = register_module("conv2", conv2);
down2 = register_module("down2", down2);
conv3 = register_module("conv3", conv3);
down3 = register_module("down3", down3);
out_conv = register_module("out_conv", out_conv);
}
torch::Tensor plainCNN::forward(torch::Tensor x)
{
x = conv1->forward(x);
cout << x.sizes() << endl;
x = down1->forward(x);
cout << x.sizes() << endl;
x = conv2->forward(x);
cout << x.sizes() << endl;
x = down2->forward(x);
cout << x.sizes() << endl;
x = conv3->forward(x);
cout << x.sizes() << endl;
x = down3->forward(x);
cout << x.sizes() << endl;
x = out_conv->forward(x);
cout << x.sizes() << endl;
return x;
}
结果
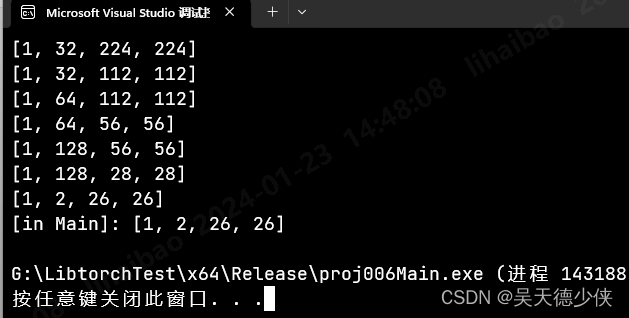