这里写目录标题
- export-scn.py
- 加载模型
- 设置每层的精度属性
- 初始化输入参数
- 导出模型
- model.encoder_layers 设置
- 初始化参数
- 设置 indice_key 属性
- 更改 lidar backbone 的 forward
- 更改lidar网络内各个层的forward
- 带参数装饰器,钩子函数代码
- 使用装饰器修改forward举例
- 跟踪模型推理
- 初始化张量
- lidar.backbone 前向操作
- conv_input
- conv_input.forward
- conv_input.TensorQuantizer.forward
- spconv.conv.SparseConvolution.forward
- symbolic_sparse_convolution_quant
- spconv.conv.SparseConvolution.forward
- encoder_layers
- 结构介绍
- 前向操作
- SparseBasicBlock.forward
- SparseConvolutionQuant.forward
- QuantAdd.forward
- ReLU.forward
- 存储 lidar.backbone.encoder_layers 每一层的前向结果
- conv_output
- Dense 操作
- Permute 和 Reshape 操作
- 打印出的节点信息
- 构建输入输出
- 构建计算图
- 构建模型
- 清空内存
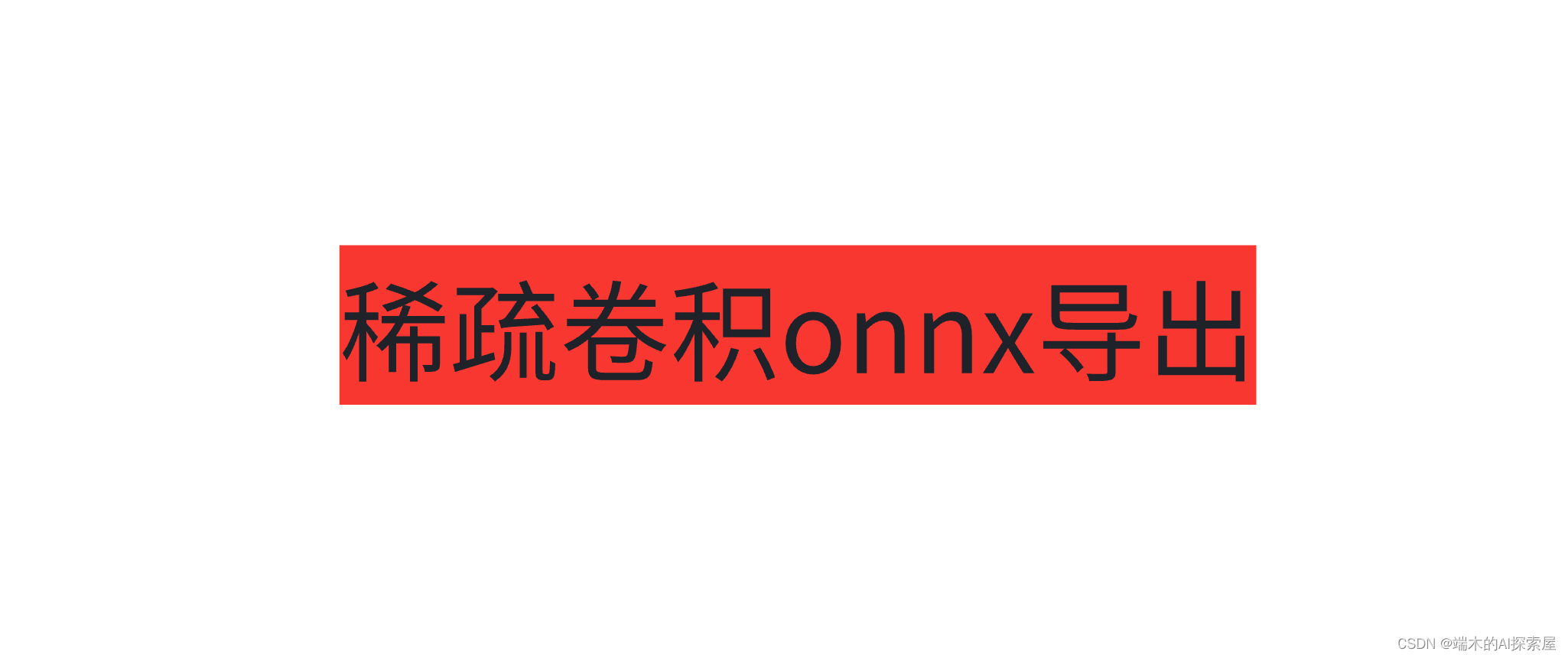
稀疏卷积网络导出onnx,是一个难点,CUDA_BEVFusion提供了详尽的导出方式。
export-scn.py
-
主要目的:该python脚本主要负责导出onnx模型。
-
难点见下方:
- 无法直接导出onnx,需要自定义导出onnx。
- 因此需要实现trace,在不影响原先模型forward的功能前提下,直接替换成我们自己的forward。
- 需要使用onnx helper创建节点。
- 无法直接导出onnx,需要自定义导出onnx。
-
流程
-
helper.make_node
- 官网链接:https://onnx.ai/onnx/api/helper.html#onnx.helper.make_node
- 作用:Construct a NodeProto.
- 解释:创建node节点,例如常见的Conv、不常见的自定义SparseConvolution
- 图片举例:
-
helper.make_tensor
- 官网链接:https://onnx.ai/onnx/api/helper.html#onnx.helper.make_tensor
- 作用:Make a TensorProto with specified arguments.
- 解释:创建initializer,例如Conv的weight或者bias
- 图片举例
-
helper.make_value_info
-
官网链接:https://onnx.ai/onnx/api/helper.html#onnx.helper.make_value_info
-
作用:Makes a ValueInfoProto with the given type_proto.
-
解释:inputs或者outputs节点属于 ValueInfoProto
-
图示举例
-
make_tensor_type_proto 根据类型和数据形状创建TypeProto
-
-
helper.make_graph
- 官网链接:https://onnx.ai/onnx/api/helper.html#onnx.helper.make_graph
- 作用:Construct a GraphProto
- 解释:用上面构建的所有NodeProto、TensorProto、ValueInfoProto
- 图片举例
-
helper.make_operatorsetid
-
官网链接:https://onnx.ai/onnx/api/helper.html#onnx.helper.make_operatorsetid
-
作用:Construct an OperatorSetIdProto.
-
解释(暂不确定):涉及ONNX 中的 “域”(domain)的概念,“ai.onnx.ml” 这样的字符串,代表了特定的组织或功能集。较为固定,这么写就行,不要改动
-
图片实例
-
GPT回答
onnx.helper.make_operatorsetid
是一个辅助函数,用于创建一个OperatorSetIdProto
对象。在 ONNX 中,OperatorSetIdProto
表示特定域的操作集的版本。要理解其作用,我们需要首先了解 ONNX 中的 “域”(domain)和 “操作集”(operator set)的概念:
-
域 (Domain): 域是一个组织或实体,它定义了一组操作。默认的域是 “”(空字符串),这是 ONNX 定义的标准操作集。其他域可以是像 “Microsoft”、“ai.onnx.ml” 这样的字符串,代表了特定的组织或功能集。
-
操作集 (Operator Set): 操作集是一组操作的集合,每个操作定义了其输入、输出和计算的语义。操作集有版本,因为随着时间的推移,域中的操作可能会增加、修改或删除。
OperatorSetIdProto
用于指定特定域中的特定版本的操作集。这是 ONNX 模型元数据的一部分,确保模型可以在理解这些操作的后端上正确运行。当你看到
onnx.helper.make_operatorsetid
这样的函数,其目的是为给定的域和版本号构建一个OperatorSetIdProto
对象。举个例子,如果你的模型使用了 ONNX 的标准操作集中的操作,并且这些操作来自版本9的操作集,那么你会这样使用这个函数:
-
-
-
opset_id = onnx.helper.make_operatorsetid("", 9)
这指示模型使用的操作来自于默认域(ONNX 标准操作集)的版本9。这样,当模型在不同的后端上加载时,后端可以确保它支持所需的操作版本。
-
helper.make_model
- 官网链接:https://onnx.ai/onnx/api/helper.html#onnx.helper.make_model
- 作用:Construct a ModelProto
- 解释:创建一个graph
- 图片举例
-
onnx.save_model
最后是保存模型的API。
加载模型
- 与导出 camera 模型类似,但是使用了 half (fl16) 精度,并将模型中所有的 TensorQuantizer 的 _disable 设置为 True,这样模型前向时就不会进行量化的前向操作。
- 注意这里使用了 half,是因为 spconv 支持 fp16 推理,性能比较好。
设置每层的精度属性
在每层添加了 precision 和 output_precision 属性,值为 “int8”,然后将第一个卷积的输入精度和最后一个卷积的输出精度设置为了 “fp16”。
初始化输入参数
voxles 和 coors 为稀疏卷积的输入参数,voxel 中储存了具体的值,形状为 [1, 5],coors 中储存了体素的位置。
导出模型
- 以下是导出模型的思路。对于spconv这种特殊的网络,nvidia选择了使用自己自定义onnx的方式,配合自己的动态库进行推理。
导出onnx功能写在了下方的函数中
model.encoder_layers 设置
- 原始的 encoder_layers 结构如下:
(encoder_layers): SparseSequential(
(encoder_layer1): SparseSequential(
(0): SparseBasicBlock(
(conv1): SubMConv3d()
(bn1): BatchNorm1d(16, eps=0.001, momentum=0.01, affine=True, track_running_stats=True)
(conv2): SubMConv3d()
(bn2): BatchNorm1d(16, eps=0.001, momentum=0.01, affine=True, track_running_stats=True)
(relu): ReLU(inplace=True)
)
(1): SparseBasicBlock(
(conv1): SubMConv3d()
(bn1): BatchNorm1d(16, eps=0.001, momentum=0.01, affine=True, track_running_stats=True)
(conv2): SubMConv3d()
(bn2): BatchNorm1d(16, eps=0.001, momentum=0.01, affine=True, track_running_stats=True)
(relu): ReLU(inplace=True)
)
(2): SparseSequential(
(0): SparseConv3d()
(1): BatchNorm1d(32, eps=0.001, momentum=0.01, affine=True, track_running_stats=True)
(2): ReLU(inplace=True)
)
)
(encoder_layer2): SparseSequential(
(0): SparseBasicBlock(
(conv1): SubMConv3d()
(bn1): BatchNorm1d(32, eps=0.001, momentum=0.01, affine=True, track_running_stats=True)
(conv2): SubMConv3d()
(bn2): BatchNorm1d(32, eps=0.001, momentum=0.01, affine=True, track_running_stats=True)
(relu): ReLU(inplace=True)
)
(1): SparseBasicBlock(
(conv1): SubMConv3d()
(bn1): BatchNorm1d(32, eps=0.001, momentum=0.01, affine=True, track_running_stats=True)
(conv2): SubMConv3d()
(bn2): BatchNorm1d(32, eps=0.001, momentum=0.01, affine=True, track_running_stats=True)
(relu): ReLU(inplace=True)
)
(2): SparseSequential(
(0): SparseConv3d()
(1): BatchNorm1d(64, eps=0.001, momentum=0.01, affine=True, track_running_stats=True)
(2): ReLU(inplace=True)
)
)
(encoder_layer3): SparseSequential(
(0): SparseBasicBlock(
(conv1): SubMConv3d()
(bn1): BatchNorm1d(64, eps=0.001, momentum=0.01, affine=True, track_running_stats=True)
(conv2): SubMConv3d()
(bn2): BatchNorm1d(64, eps=0.001, momentum=0.01, affine=True, track_running_stats=True)
(relu): ReLU(inplace=True)
)
(1): SparseBasicBlock(
(conv1): SubMConv3d()
(bn1): BatchNorm1d(64, eps=0.001, momentum=0.01, affine=True, track_running_stats=True)
(conv2): SubMConv3d()
(bn2): BatchNorm1d(64, eps=0.001, momentum=0.01, affine=True, track_running_stats=True)
(relu): ReLU(inplace=True)
)
(2): SparseSequential(
(0): SparseConv3d()
(1): BatchNorm1d(128, eps=0.001, momentum=0.01, affine=True, track_running_stats=True)
(2): ReLU(inplace=True)
)
)
(encoder_layer4): SparseSequential(
(0): SparseBasicBlock(
(conv1): SubMConv3d()
(bn1): BatchNorm1d(128, eps=0.001, momentum=0.01, affine=True, track_running_stats=True)
(conv2): SubMConv3d()
(bn2): BatchNorm1d(128, eps=0.001, momentum=0.01, affine=True, track_running_stats=True)
(relu): ReLU(inplace=True)
)
(1): SparseBasicBlock(
(conv1): SubMConv3d()
(bn1): BatchNorm1d(128, eps=0.001, momentum=0.01, affine=True, track_running_stats=True)
(conv2): SubMConv3d()
(bn2): BatchNorm1d(128, eps=0.001, momentum=0.01, affine=True, track_running_stats=True)
(relu): ReLU(inplace=True)
)
)
初始化参数
- model中有的属性概览
设置 indice_key 属性
-
没量化前的model.encoder_layers特点:
- encoder_layer1~4中,0和1的模块里面的con1、conv2的稀疏卷积都是SubM的
-
添加属性:按 layer 的索引来给每个子模块添加属性,可以通过这个属性来判断稀疏卷积属于哪一层。
更改 lidar backbone 的 forward
更改后的
- 此时返回的是impl函数。impl函数内的代码还没有执行
原本的 forward
修改前后的主要的区别在于添加了一个对于 inverse_indices 的判断,对特征是XYZ的顺序,还是ZYX的顺序都支持。
更改lidar网络内各个层的forward
https://ke.qq.com/course/5851686#term_id=106187062
带参数装饰器,钩子函数代码
- 了解如何更改forward,以及如何注册自定义onnx的node。需要先了解前面的带参装饰器作用。
使用装饰器修改forward举例
跟踪模型推理
初始化张量
voxels即我们创建的全是0的,形状为torch.Size([1, 5])的张量。
往34行定义好的,全局变量obj_to_tensor_id
添加键值。
-
obj_to_tensor_id
中
- key:将 obj (即 voxel) 的id作为 key
- value:obj_to_tensor_id
的长度作为value -
如果obj是
SparseConvTensor
的话,使用SparseConvTensor
的features的id作为标记。- 因为SparseConvTensor只是个壳子,说明它的地址是可能被复用的。但是
SparseConvTensor.features
对象是我们需要的,features地址是唯一的。需要获取他的id。
- 因为SparseConvTensor只是个壳子,说明它的地址是可能被复用的。但是
-
另一个解释
- obj_to_tensor_id 的主要作用是用于后续查看每个模块的输入输出时可以通过更加直观的有序的数字来表示,也方便确认节点是否正确。
- enable_trace 作为一个开关,给后续使用。这里设置为True。
- 真正使用的地方在
- 主要用途是作为一个标记,避免在调用old
lidar.backbone 前向操作
- 可以先看
workspace/12Explain_onnx/02hook2.ipynb
-
根据 model 刚才更新的 forward 进行前向,主要分为三个部分,分别是
- conv_input
- encoder_layers
- conv_out
-
首先需要将 coors 更改为 int 类型(271行),然后通过特征值、索引、空间形状、批次构建一个 SparseTensor 对象作为输入。
-
在前向过程中,会发现** model 内部的子模块的 forward 被替换了**,是因为装饰器将原本的 forward 的内容替换了。register_node 装饰器被用来替换稀疏卷积网络的 forward 函数,然后在被它装饰的函数中进行前向操作的同时记录了 onnx 节点 (node) 和初始化器 (initializer)。在生成计算图时,这些记录的信息会被用于创建 onnx 模型。
conv_input
- 第一个部分的前向
conv_input.forward
oldfn(self, *args) 相当于 SparseConvolutionQuant.forward(model.conv_input, SparseConvTensor)
,会调用在 quantize.py 中自定义的 SparseConvolutionQuant
中的 forward
方法。
- oldfn就是原本的forward
,计算结果赋值给y,最后返回的也是这个y
-
400行全局变量enable_trace的会被置为True表示开启追踪,60行会置为False。表示旧的forward即oldfn内的前向,不会进行后续的数据记录的操作,因为后续会将 SparseConvolutionQuant 当作一个节点,内部的 SparseConvolution 只是一个计算步骤,不会单独作为一个节点。
注意断点不要搭载52、53行。打在61行好调试
新加52行的打印信息后打印的信息。conv_input的卷积是subm卷积。输入输出都被正常追踪。
conv_input.TensorQuantizer.forward
由于之前已经将整个lidar.backbone 中的 TensorQuantizer 的 _disabled 属性设置为了 True,所以这里并不会对输入和权重进行量化。
spconv.conv.SparseConvolution.forward
原来这里应该调用 spconv.conv.SparseConvolution.forward
方法,也被替换为了 internal_forward
。
因为之前将全局变量 enable_trace 修改为了 False,这里会调用 SparseConvolution.forward。当前的稀疏卷积为 SubMConv3d 的对象。
symbolic_sparse_convolution_quant
这样对于原本模块的前向操作就全部完成了,会回到最外层的 internal_forward 函数中继续之后的操作。
节点信息
入参:ilayer 为当前 layer 的索引;y 为当前模块原本的前向结果;x 为当前模块的输入。
-
先将模块的前向结果注册到 obj_to_tensor_id 中,y 是 SparseConvTensor,所以会将 id(y.features) 作为 key。
-
之后根据模块的输入特征尺度,核大小,步长,填充和膨胀来计算输出的尺寸。
-
这里使用的是 SubMConv3d,要保持输入个数与输出个数相同。
-
将输出的通道数修改为模块中指定的数量。
-
使用 list 来储存当前输入的信息,其中第一个元素是输入的特征 x.features 的 id 对应的转换为 string 的索引 obj_to_tensor_id[id(x.features)],第二个元素中调用了 torch.Tensor.permute 函数,会被替换为 internal_forward,由于 enable_trace 为 False,还是执行原来的 torch.Tensor.permute 函数来调整权重的维度,从 KIO 变换为 OKI,最终第二个元素为 “spconv0.weight” (append_initializer 函数介绍)。如果当前模块有 bias 的话,就会将 “spconv0.bias” 作为第三个元素,在这里是具有 bias 的,会在 inputs 和 initializers 中添加对应的数据。
-
最后在 nodes 中添加一个 NodeProto 对象,这个节点记录了当前稀疏卷积模块的操作 (make_node 函数介绍)。
spconv.conv.SparseConvolution.forward
这里 bind_fn 函数就执行完毕了,后续将 enable_trace 改为 True,后续就可以继续添加节点和计算图信息。之后将使用过的输入和输出存储在 avoid_reuse_container 数组中 (用于防止重复使用)。然后将局部变量 ilayer 添加 1,主要用于表示当前操作的索引,这样节点的名称就是按顺序的并且不会重复。如果当前的 SparseConvolutionQuant 是 lidar.backbone.encoders 中第 4 个进行前向的 SparseConvolutionQuant,那么 ilayer 就是 3,节点的名称为 conv3。最后返回前向的结果。
节点信息
encoder_layers
- 代码
SparseSequential(
(encoder_layer1): SparseSequential(
(0): SparseBasicBlock(
(conv1): SparseConvolutionQunat(
(_input_quantizer): TensorQuantizer(disabled)
(_weight_quantizer): TensorQuantizer(disabled)
)
(conv2): SparseConvolutionQunat(
(_input_quantizer): TensorQuantizer(disabled)
(_weight_quantizer): TensorQuantizer(disabled)
)
(relu): ReLU()
(quant_add): QuantAdd(
(_input_quantizer): TensorQuantizer(disabled)
)
)
(1): SparseBasicBlock(
(conv1): SparseConvolutionQunat(
(_input_quantizer): TensorQuantizer(disabled)
(_weight_quantizer): TensorQuantizer(disabled)
)
(conv2): SparseConvolutionQunat(
(_input_quantizer): TensorQuantizer(disabled)
(_weight_quantizer): TensorQuantizer(disabled)
)
(relu): ReLU()
(quant_add): QuantAdd(
(_input_quantizer): TensorQuantizer(disabled)
)
)
(2): SparseConvolutionQunat(
(_input_quantizer): TensorQuantizer(disabled)
(_weight_quantizer): TensorQuantizer(disabled)
)
)
(encoder_layer2): SparseSequential(
(0): SparseBasicBlock(
(conv1): SparseConvolutionQunat(
(_input_quantizer): TensorQuantizer(disabled)
(_weight_quantizer): TensorQuantizer(disabled)
)
(conv2): SparseConvolutionQunat(
(_input_quantizer): TensorQuantizer(disabled)
(_weight_quantizer): TensorQuantizer(disabled)
)
(relu): ReLU()
(quant_add): QuantAdd(
(_input_quantizer): TensorQuantizer(disabled)
)
)
(1): SparseBasicBlock(
(conv1): SparseConvolutionQunat(
(_input_quantizer): TensorQuantizer(disabled)
(_weight_quantizer): TensorQuantizer(disabled)
)
(conv2): SparseConvolutionQunat(
(_input_quantizer): TensorQuantizer(disabled)
(_weight_quantizer): TensorQuantizer(disabled)
)
(relu): ReLU()
(quant_add): QuantAdd(
(_input_quantizer): TensorQuantizer(disabled)
)
)
(2): SparseConvolutionQunat(
(_input_quantizer): TensorQuantizer(disabled)
(_weight_quantizer): TensorQuantizer(disabled)
)
)
(encoder_layer3): SparseSequential(
(0): SparseBasicBlock(
(conv1): SparseConvolutionQunat(
(_input_quantizer): TensorQuantizer(disabled)
(_weight_quantizer): TensorQuantizer(disabled)
)
(conv2): SparseConvolutionQunat(
(_input_quantizer): TensorQuantizer(disabled)
(_weight_quantizer): TensorQuantizer(disabled)
)
(relu): ReLU()
(quant_add): QuantAdd(
(_input_quantizer): TensorQuantizer(disabled)
)
)
(1): SparseBasicBlock(
(conv1): SparseConvolutionQunat(
(_input_quantizer): TensorQuantizer(disabled)
(_weight_quantizer): TensorQuantizer(disabled)
)
(conv2): SparseConvolutionQunat(
(_input_quantizer): TensorQuantizer(disabled)
(_weight_quantizer): TensorQuantizer(disabled)
)
(relu): ReLU()
(quant_add): QuantAdd(
(_input_quantizer): TensorQuantizer(disabled)
)
)
(2): SparseConvolutionQunat(
(_input_quantizer): TensorQuantizer(disabled)
(_weight_quantizer): TensorQuantizer(disabled)
)
)
(encoder_layer4): SparseSequential(
(0): SparseBasicBlock(
(conv1): SparseConvolutionQunat(
(_input_quantizer): TensorQuantizer(disabled)
(_weight_quantizer): TensorQuantizer(disabled)
)
(conv2): SparseConvolutionQunat(
(_input_quantizer): TensorQuantizer(disabled)
(_weight_quantizer): TensorQuantizer(disabled)
)
(relu): ReLU()
(quant_add): QuantAdd(
(_input_quantizer): TensorQuantizer(disabled)
)
)
(1): SparseBasicBlock(
(conv1): SparseConvolutionQunat(
(_input_quantizer): TensorQuantizer(disabled)
(_weight_quantizer): TensorQuantizer(disabled)
)
(conv2): SparseConvolutionQunat(
(_input_quantizer): TensorQuantizer(disabled)
(_weight_quantizer): TensorQuantizer(disabled)
)
(relu): ReLU()
(quant_add): QuantAdd(
(_input_quantizer): TensorQuantizer(disabled)
)
)
)
)
结构介绍
encoder_layers 中包含了 4 个 layer,前 3 个 layer 包含了 2 个 SparseBasicBlock 和 1 个 SparseConvolutionQuant (sparseconv 融合了 bn 和 relu,原先是一个 SparseSequential),最后 1 个 layer 仅包含 2 个 SparseBasicBlock。SparseBasicBlock 内部就是 2 个 SparseConvolutionQuant 和 ReLU。
前向操作
SparseBasicBlock.forward
遍历 lidar.backbone.encoder_layers 中的所有 layer,将之前 conv_input 的前向结果作为第一个 layer 的输入进行前向操作。
第一个layer中的结构,后续以这个layer进行介绍
这里会遍历刚才 layer 中的每个子模块并进行前向操作,当前的子模块为第一个 SparseBasicBlock,这个模块的前向函数在量化时被替换了。
forward 被替换为了 new_sparse_basic_block_forward 对象,在调用 forward 时,就是调用这里的 __call__函数。当前的 self.is_fuse_relu 是 True,因为在量化时有融合 ReLU 的操作。
节点信息
SparseConvolutionQuant.forward
self.conv1 和 self.conv2 的前向与 conv_input 的前向是相同的,就不重复介绍了,看一下 nodes、initializers、obj_to_tensor_id 和 avoid_reuse_container。
QuantAdd.forward
这里会进行 quant_add 前向 (残差操作),它的前向也被替换为了 internal_forward,总体流程与上面的稀疏卷积的相同。
-
调用 quant_add.forward 获得前向的结果 (torch.add)
-
调用 symbolic_add_quant 注册前向的结果和添加节点信息
- https://onnx.ai/onnx/api/helper.html#onnx.helper.make_node make_node 官网
- https://onnx.ai/onnx/operators/onnx__Add.html#l-onnx-doc-add add 官网
-
填充输入和输出到 avoid_reuse_container(internal_forward 中 65 行)
-
更新 SparseConvTensor 的 feature(125 行)
- 每个 SparseConvTensor 的 features 相加。然后再构建成新的 SparseConvTensor,赋值给 out
- 每个 SparseConvTensor 的 features 相加。然后再构建成新的 SparseConvTensor,赋值给 out
ReLU.forward
ReLU 的前向也被替换为了 internal_forward。
之后会继续遍历子模块,如果属于 SparseModule,那么前向流程就是上面这些步骤,因为这里的模块只有 SparseBasicBlock 和 SparseConvolutionQuant,它们都属于 SparseModule,之后遍历子模块的前向流程都是相同的。
存储 lidar.backbone.encoder_layers 每一层的前向结果
节点信息
conv_output
这个模块与 conv_input 相同,它们的流程是一样的。
Dense 操作
dense 也被替换为了 internal_forward,看一下原来的 dense 内部是怎么运作的。
-
计算输出的形状 (1, 180, 180, 2, 128)。
-
根据输出形状构建一个全 0 张量,并将前向结果根据索引 (0, 0, 0, 0) 填充到这个张量中。
- ndim=4
- 13 行 output_shape 变为[1, 128],<class ‘list’>
- 15 行[tensor([0], device=‘cuda:0’), tensor([0], device=‘cuda:0’), tensor([0], device=‘cuda:0’), tensor([0], device=‘cuda:0’)]
ellipsis
是一个内置的对象,通常表示为三个连续的点...
-
调整将结果的维度顺序 ([0, 4, 1, 2, 3], N X Y Z C -> N C X Y Z)。(59 行)
-
创建 onnx 的 node 节点。自定义节点。
op_type设置为了ScatterDense
Permute 和 Reshape 操作
调整输出的维度顺序 (0, 1, 4, 2, 3),从 N C X Y Z -> N C Z X Y,之后通过 reshape 将 C 和 Z 合并在一起,permute 和 reshape 函数都会被替换为 interval_forward,之后会将这两个操作添加到节点中。Permute 操作对应节点的 op_type 为 Transpose。
# 填充features并permute和reshape后的数值
for i in range(0, 256, 2):
print(spatial_features[0][i][0][0])
打印出的节点信息
--> SparseConvolutionQunat0[subm] -> Input 0, Output 1
--> SparseConvolutionQunat1[subm] -> Input 1, Output 2
--> SparseConvolutionQunat2[subm] -> Input 2, Output 3
--> QuantAdd0 -> Input 3 + 1, Output 4
--> ReLU0 -> Input 4, Output 5
--> SparseConvolutionQunat3[subm] -> Input 5, Output 6
--> SparseConvolutionQunat4[subm] -> Input 6, Output 7
--> QuantAdd1 -> Input 7 + 5, Output 8
--> ReLU1 -> Input 8, Output 9
--> SparseConvolutionQunat5[conv] -> Input 9, Output 10
--> SparseConvolutionQunat6[subm] -> Input 10, Output 11
--> SparseConvolutionQunat7[subm] -> Input 11, Output 12
--> QuantAdd2 -> Input 12 + 10, Output 13
--> ReLU2 -> Input 13, Output 14
--> SparseConvolutionQunat8[subm] -> Input 14, Output 15
--> SparseConvolutionQunat9[subm] -> Input 15, Output 16
--> QuantAdd3 -> Input 16 + 14, Output 17
--> ReLU3 -> Input 17, Output 18
--> SparseConvolutionQunat10[conv] -> Input 18, Output 19
--> SparseConvolutionQunat11[subm] -> Input 19, Output 20
--> SparseConvolutionQunat12[subm] -> Input 20, Output 21
--> QuantAdd4 -> Input 21 + 19, Output 22
--> ReLU4 -> Input 22, Output 23
--> SparseConvolutionQunat13[subm] -> Input 23, Output 24
--> SparseConvolutionQunat14[subm] -> Input 24, Output 25
--> QuantAdd5 -> Input 25 + 23, Output 26
--> ReLU5 -> Input 26, Output 27
--> SparseConvolutionQunat15[conv] -> Input 27, Output 28
--> SparseConvolutionQunat16[subm] -> Input 28, Output 29
--> SparseConvolutionQunat17[subm] -> Input 29, Output 30
--> QuantAdd6 -> Input 30 + 28, Output 31
--> ReLU6 -> Input 31, Output 32
--> SparseConvolutionQunat18[subm] -> Input 32, Output 33
--> SparseConvolutionQunat19[subm] -> Input 33, Output 34
--> QuantAdd7 -> Input 34 + 32, Output 35
--> ReLU7 -> Input 35, Output 36
--> SparseConvolutionQunat20[conv] -> Input 36, Output 37
--> ToDense0[[180, 180, 2]][[1, 128, 180, 180, 2]] -> Input 37, Output 38
--> Permute0[(0, 1, 4, 2, 3)][[1, 128, 2, 180, 180]] -> Input 38, Output 39
--> Reshape0[(1, 256, 180, 180)] -> Input 39, Output 40
构建输入输出
通过 make_value_info 来构建一个 ValueInfoProto 对象,name 是一个 str,用于对应第一个节点中的输入名称,type_proto 是一个 TypeProto 对象,用于描述数据类型和形状。这里生成的 inputs 和 outputs 用于作为后面生成计算图时的输入和输出。
构建计算图
通过 make_graph 来构建一个 GraphProto 对象,name 是一个 str,inputs 和 outpus 就是一个类型为 TensorProto 的 list,nodes 是一个类型为 NodeProto 的 list,initializer 是一个类型为 TensorProto 的 list,通过这些信息就可以构建一个计算图。
构建模型
通过 make_model 来构建一个 ModelProto 对象,graph 是一个 GraphProto 对象,后续的参数为关键词参数。一个模型对应一个计算图,opset 中的 domain 设置为了 ai.onnx 表示操作集所属的域,version 设置为了 11 表示操作集的版本号,这两个参数要支持之前在节点中使用的算子。